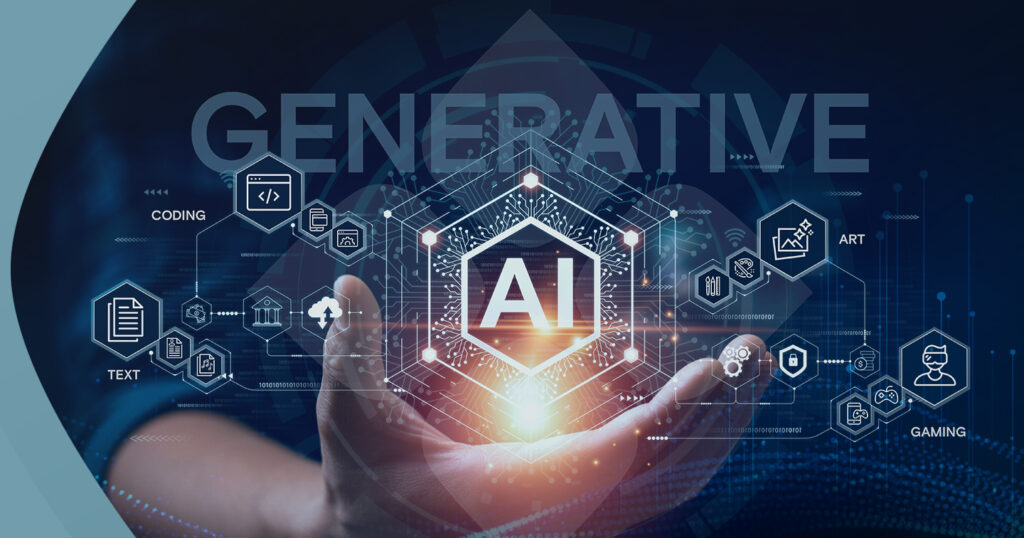
Generative AI, a rapidly advancing subset of artificial intelligence, has revolutionized how enterprises approach creativity, efficiency, and problem-solving. From generating realistic images and videos to crafting coherent text and code, generative AI models like GPT-4, DALL-E, and Stable Diffusion offer unprecedented capabilities that can transform industries. While the potential is significant, implementing generative AI in enterprise settings also brings notable risks that businesses must navigate carefully. Here’s a closer look at the opportunities and risks associated with generative AI in the enterprise.
Thank you for reading this post, don't forget to subscribe!Opportunities of Generative AI in the Enterprise
Enhanced Content Creation and Marketing
Generative AI is a powerful tool for automating content creation, enabling companies to produce high-quality marketing materials, social media content, and personalized messages quickly and efficiently. AI can generate blog posts, social media posts, images, and videos based on a company’s style and target audience, saving marketing teams time while boosting creativity. Additionally, tools like ChatGPT can automate customer engagement by handling queries, providing recommendations, and supporting lead generation.
Example: Jasper.ai, a generative AI tool, assists businesses in generating marketing copy that aligns with brand voice, making it easier to execute campaigns without large content teams.Automated Software Development and Testing
Generative AI models like GitHub Copilot and OpenAI’s Codex offer automated code generation, which accelerates software development by assisting developers with code snippets, debugging, and even full-function implementations. These tools improve productivity and allow developers to focus on higher-level design and problem-solving. For quality assurance, generative AI can assist in creating unit tests, identifying vulnerabilities, and performing code reviews, which strengthens the reliability of software products.
Example: GitHub Copilot has become a popular tool among developers, helping them reduce coding time by suggesting relevant code snippets and automating repetitive tasks.Advanced Data Synthesis for Insights and Analysis
Generative AI can simulate data sets for training machine learning models when real data is unavailable or insufficient. This synthesized data can mimic real-world patterns without compromising sensitive information, making it useful for industries like healthcare and finance that require strict data privacy. Additionally, generative models can help businesses generate insights by summarizing large volumes of data and highlighting patterns that might otherwise go unnoticed.
Example: Financial institutions are leveraging generative AI to synthesize customer data for training fraud detection models, preserving privacy while improving accuracy.Personalized Customer Experiences
Generative AI allows for the creation of personalized user experiences through chatbots, virtual assistants, and recommendation engines. AI-powered chatbots can handle personalized interactions at scale, from product recommendations to troubleshooting support. By analyzing customer preferences and behaviors, generative AI can create tailored content and product suggestions, enhancing the customer experience and improving retention rates.
Example: E-commerce platforms use AI to suggest products based on a customer’s previous searches and purchases, making the shopping experience more intuitive and engaging.Design and Prototyping
Generative AI is streamlining design processes by helping companies quickly create and visualize prototypes. Tools like DALL-E enable designers to generate multiple design concepts, mock-ups, and prototypes based on text prompts, expediting the design process. This capability is especially valuable for industries like fashion, product design, and architecture, where rapid prototyping is crucial to staying competitive.
Example: A furniture manufacturer might use AI-generated designs to explore new ideas quickly, allowing them to bring innovative products to market faster.
Risks of Generative AI in the Enterprise
Data Privacy and Compliance Challenges
Generative AI models require vast amounts of data to function effectively, and this often includes sensitive information. Data privacy regulations like GDPR, CCPA, and HIPAA place stringent requirements on data handling, and any misuse of personal data by AI can result in serious compliance issues. Additionally, generative models trained on public data may inadvertently produce outputs containing sensitive or proprietary information, posing a risk to privacy.
Risk Management: To mitigate this, companies should implement strict data anonymization practices, use synthetic data where possible, and monitor AI outputs to ensure compliance with data protection laws.Intellectual Property and Copyright Issues
Generative AI can create content that closely resembles or even replicates existing works, leading to intellectual property concerns. Companies that deploy generative AI to create branded content may face legal challenges if the AI-generated work is found to infringe on copyrighted material. This risk becomes even greater when the AI is trained on publicly available datasets that include copyrighted content.
Risk Management: Enterprises should prioritize transparency regarding the data sources used for training AI models and consult with legal experts to ensure compliance with intellectual property laws. Tools that verify the originality of AI-generated content can also help reduce these risks.Ethical and Bias Concerns
Generative AI models can unintentionally reproduce biases present in their training data, leading to ethical concerns and potential reputational damage. For instance, an AI model used for hiring recommendations might exhibit bias based on gender or ethnicity if it was trained on biased data. This can lead to biased hiring decisions, customer service interactions, or marketing content that alienates certain groups.
Risk Management: To address bias, companies should implement AI auditing practices, review training data for fairness, and continuously monitor AI outputs. Additionally, using diverse data sources and regularly updating models can help minimize bias.Misinformation and Brand Safety Risks
Generative AI models can produce content that sounds credible but is factually incorrect, which poses a risk for enterprises that rely on AI for content generation or customer interactions. If left unchecked, this misinformation can damage a company’s reputation and lead to the spread of inaccurate information. Additionally, when AI is used to create branded content, there’s a risk that it could produce outputs that do not align with the brand’s values.
Risk Management: Organizations should implement validation steps, requiring human oversight to review AI-generated content before publishing. This ensures factual accuracy and alignment with brand standards.Security Vulnerabilities
Generative AI models, particularly those deployed in customer-facing applications, can expose enterprises to cybersecurity risks. For instance, generative models used in automated customer support could be exploited to extract sensitive information. Additionally, if an AI model is manipulated to generate malicious content, it could harm both the company and its customers.
Risk Management: Businesses should apply robust cybersecurity practices, including regular model testing and monitoring, access control measures, and user authentication, to safeguard against potential vulnerabilities.
Best Practices for Safe and Effective Use of Generative AI in the Enterprise
Human-in-the-Loop (HITL) Approach: Involve human oversight in AI processes to monitor outputs, validate quality, and manage risks. This ensures that AI-generated content is accurate and aligns with brand guidelines.
Regular Model Audits: Conduct frequent audits of AI models to assess for biases, vulnerabilities, and adherence to legal standards. Model auditing is especially important in sectors like finance, healthcare, and hiring, where ethics and compliance are paramount.
Transparency and Explainability: Strive for transparency by informing users about the role of AI in content generation. Explainable AI methods help users understand how AI models make decisions, increasing trust and accountability.
Data Privacy and Security Compliance: Ensure that AI models handle data securely and comply with regulatory requirements. Regularly update privacy policies and practices to address any emerging threats.
Define Clear Usage Policies: Establish guidelines that define when and how generative AI can be used. These policies should specify acceptable use cases, ethical considerations, and limitations, especially when dealing with sensitive data or customer-facing content.
Conclusion
Generative AI has transformative potential for enterprises, offering exciting opportunities for productivity, innovation, and enhanced customer engagement. However, as organizations integrate this technology, they must be mindful of the associated risks, from data privacy and compliance to ethical and security challenges. By implementing best practices and adopting a cautious, informed approach, businesses can harness generative AI’s capabilities effectively while minimizing potential downsides. As generative AI continues to evolve, a balanced approach will enable organizations to leverage this technology for a competitive edge in a responsible and sustainable way.